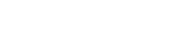
An essential step of risk assessment methodologies in subsurface activities is to simulate multiple possible realizations for the spatial distribution of the lithological facies.
However, facies simulations can be peculiarly demanding in contexts where the facies distribution is strongly controlled by the location and shape of specific objects. A typical example is fluvial deposition environments, where the facies distribution is tightly linked to the features of the past river channels. In this example, all standard geostatistical approaches imply a difficult tradeoff between conditioning the simulation to the available well data and generating channels which are geologically realistic.
Thus, in this project, we explored an emerging approach to facies simulation, leveraging Generative Artificial Intelligence techniques. We appraised its merits to conciliate hard-data conditioning with geological realism.
Practically, we trained Self-Attention Generative Adversarial Networks (SAGAN) on three public datasets, corresponding to various types of fluvial environments, and assessed the results using a set of 12 advanced geological metrics.
Our results highlighted the promises of the approach, as the SAGANs are able to satisfactorily reproduce the geological features of the channels learned from the training images, while respecting the well observations.
This propitious proof of concept paves the way to future integration of the technology in industrial geostatistical toolboxes, with future work focusing on increasing the flexibility of the workflow (e.g. addition of soft conditioning data such as seismic attributes).