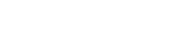
The geophysical interpretation of some key subsurface geological horizons can significantly change through time, due to different interpreters and new or reprocessed geophysical data. As such, the resulting structural maps can change accordingly. Retracing and understanding such evolution in the interpretation is very valuable for any geologist new to the field. Unfortunately, it can be peculiarly time-consuming as the various structural maps are scattered in a large number of unstructured documents of diverse types. Automatic extraction of these maps, together with the associated information, can improve the efficiency of operational workflows and help professionals save time for more advanced and higher-value activities in their daily work.
Our main objective is to present an end-to-end workflow to automate the extraction of images of interest and the associated information in geoscience documents, and its application on an operational case-study provided by an energy resources company.
We developed an integrated workflow to automate the extraction of images of interest and the associated information in geoscience documents. The implemented workflow relies on a combination of free Python packages for Natural Language Processing, Computer Vision, Optical Character Recognition and Machine Learning. This workflow was applied on a case study using data from an oil field operated by Vermilion Energy, in South-West of France.
More than 90% of the relevant images and related information were extracted from the database. Besides, the whole automated process lasted only 5 hours instead of several weeks of manual work. This illustrates the enormous gains in operational efficiency which can be unlocked with such approaches, further increased by the possibility to explore the information extracted with an interactive web interface.
The proposed workflow is not limited to the use case presented, and it can be applied with minimal adjustment to any kind of information extraction within large knowledge bases.