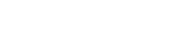
Document classification is the process of assigning categories or classes to documents to make them easier to manage, search, filter, or analyze. Traditionally, document classification is one of the major parts of the manual effort, especially when the documents to classify are scattered within a huge database.
Our objective was to develop an automatic workflow to classify documents and group them according to their topic.
We developed a workflow to classify documents, based on the similarity to the reference papers. To do so, 322 academic papers have been used and divided into 6 thematic classes: 3 geoscientific classes and 3 unrelated categories. In each category, one or more papers were set as “archetypal references”. The adopted methodology combined two concepts: Text similarity, developed in ElasticSearch under a search algorithm named “More Like This”, and “Text classification”, this latter being a Supervised Machine Learning approach.
More than 90% of papers were properly classified into their own thematic.
This methodology is promising and it’s worth to improve it by testing it with a bigger database and by adding thematic classes closer to each other (e.g.: Sedimentology and sequence stratigraphy).