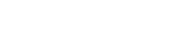
Well-log data is often the main source of information for geoscience subsurface evaluation. Nevertheless, these data have uncertainties that can induce non-negligible errors in the outcomes of machine learning workflows in which these data are used as input. The quantification of these a posteriori errors, although not always being systematically performed, can significantly benefit any decision making based on the outcomes of these machine learning workflows.
Our objective is to allow a quantification of the uncertainty in the outcomes of machine learning workflows, either in classication or in regression, induced by errors in the input log data.
Our approach first uses ‘Monte-Carlo’ type method to blurr the input log data , then quantifies the a posteriori error on output predictions of machine learning workflows both in classification and in regression.
The method is applied to wireline log data from a well Lauda1 of offshore Western Australia, for which an expert classification is exceptionally available at all the depth levels of the well. We show that the presence of error in non-spatial input data (typically physical measurements such as GR, sonic, conductivity…) induces much less uncertainty, both in classification and in regression, than the presence of errors in spatial input data (here depth).
The early results are promising and pave the way for further adaptation. The proposed method, initially intended for wireline log measurements can be adapted to interpretation data in higher dimension, i.e. maps (2D) or cubes (3D). Many such data are available in domains even outside oil & gas industry, such as in mining industry or in geothermal energy.