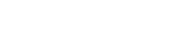
Predictive mapping enables rapid identification and targeting of areas anticipated to host important mineral deposits, thereby reducing exploration costs and time-to-discovery. However, most of the methodologies already available in the literature suffer from two major drawbacks: (i) the absence of consideration of ‘geology’ for defining the relative weights of the decision criteria (geology being integrated as a mere additional feature), and (ii) the lack of a reliability map for the prospectivity prediction.
We developed an innovative method to automatically generate maps of prospectivity, based on Machine Learning and multicriteria decision analysis. We used the public dataset from British Columbia (Canada) as case-study, with the aim to find the best sites for geothermal prospection in this region. Our method extends on a large scale the prospectivity mapping provided locally and manually by the experts, and identifies the geographical areas where the statistical approach is the least reliable.
Our method is based on multiple-criteria decision analysis (MCDA), that classically involves three main concepts: a goal (e.g. choosing the best site to prospect), different criteria (e.g. price, quality) and alternatives (e.g. multiple possible locations). Given the goal, MCDA consists in scoring the different alternatives by considering the conflicting criteria (e.g. price and quality often comes in conflict).
By applying the method to public geothermal data from British Columbia (Canada), we obtained prospectivity maps with higher spatial resolution than those from previous works. Moreover, it substantially reduces the footprint of data acquisition and facilitates the identification of geographical areas where the statistical approach is the least reliable, i.e. where the subjective human interpretation would have the greatest added value.
Our method and algorithms, notably the introduction of geology-dependent criteria weights for the decision analysis, are quickly transposable and adaptable to different subsurface exploration/exploitation contexts, for instance in the oil & gas, mining or hydrogeological industries.